Chain of Thought (CoT) prompting is an exciting innovation in the world of artificial intelligence which makes large language models (LLMs) reason better. CoT prompting simulates human‐like reasoning with step‐by‐step simulation, enabling models to address complex questions, improve their decision‐making power and produce superior and logical responses.
In this article, we explore:
- What CoT prompting is
- Its applications
- Best practices
- The future of CoT prompting
- Recent updates
- Limitations and challenges
Discover how you can leverage CoT prompting in your AI projects today.
Chain of Thought Prompting: What Is It?
Chain of Thought prompting is a method that has the language model provide the final answer after prompting, and then force it to generate a sequence of the intermediate reasoning steps. It’s similar to how humans break down their problems into small bits for the sake of clarity and precision.
Unlike traditional prompting, which often seeks direct answers, CoT prompting leverages structured reasoning paths to:
- Improve logical coherence.
- Address multi-step problems effectively.
- Enhance creative problem-solving by exploring different pathways.
For instance, a CoT-prompted model solving a math problem would outline the steps taken, making the process transparent and reducing errors.
Applications of Chain of Thought: From Logic to Creativity
1. Education and Learning
Language models using Chain of Thought can provide detailed explanations of complex concepts, making them invaluable for students and educators. Examples include:
- Step-by-step math problem-solving.
- Detailed explanations of scientific theories.
- Grammar and vocabulary breakdowns for language learning.
2. Healthcare Decision Support
CoT helps AI systems analyze symptoms and medical histories, suggesting potential conditions or treatment plans. Structured reasoning builds trust in AI for medical diagnostics.
3. Financial Analysis
By breaking down datasets and trends, CoT models assist with:
- Financial forecasting.
- Risk assessment.
- Investment strategy formulation.
4. Creative Writing and Content Generation
CoT enables AI to craft coherent stories, generate creative ideas, and structure logical arguments for essays and articles.
5. Legal and Policy Analysis
In legal contexts, Chain of Thought provides step-by-step reasoning for:
- Analyzing cases.
- Drafting policies.
- Interpreting precedents and regulations.
Best Practices for CoT Implementation
1. Provide Clear and Contextual Prompts
- Use specific examples to guide the model’s reasoning.
- Define the type of reasoning process desired.
2. Iterate and Refine
- Test variations of prompts to identify what works best.
- Validate the model’s reasoning for consistency and correctness.
3. Augment with External Knowledge
- Use domain-specific data or tools to enhance Chain of Thought.
- Employ retrieval-augmented generation (RAG) for up-to-date information.
4. Evaluate Outputs Rigorously
- Validate reasoning steps for logical consistency.
- Correct biases or errors in the thought process.
5. Visualize Complex Problems
- Represent reasoning steps with flowcharts, diagrams, or other visuals to improve interpretability.
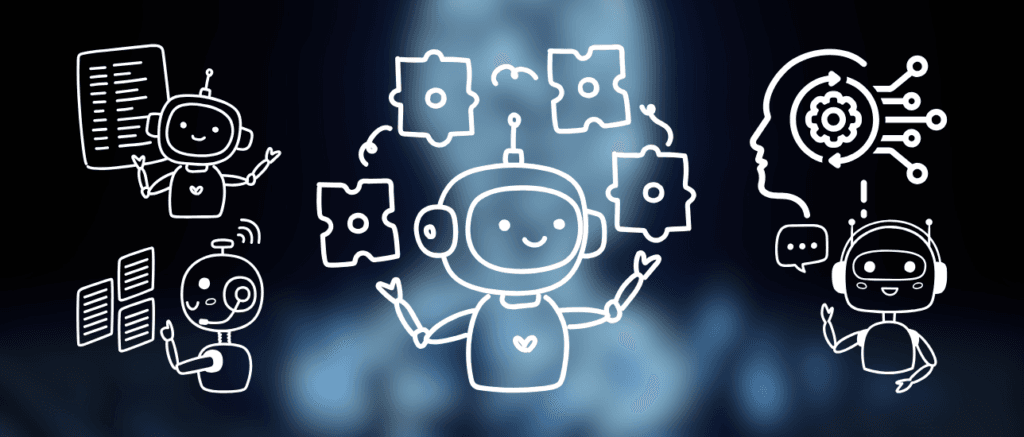
The Future of Chain of Thought in AI
1. Multimodal AI Integration
CoT reasoning may combine text, image, and speech inputs for holistic problem-solving.
2. Enhanced Personalization
AI systems can adapt CoT strategies to user-specific needs, delivering tailored solutions.
3. Synergy with Emerging Technologies
Integrating CoT with technologies like quantum computing can unlock new computational reasoning powers.
4. Advancing Education and Skill Development
Adopting CoT in educational platforms could democratize access to high-quality, step-by-step learning resources.
Chain of Thought prompting is a transformational method for improving language models’ reasoning, creativity, and decision-making abilities. Its applications span education, healthcare, finance, and beyond.
CoT 2024 updates & Developments
- Improved Accuracy: CoT prompting leads to major gains in accuracy for multi step reasoning tasks like mathematics, commonsense reasoning and symbolic manipulation. As an example, CoT prompting offered a 78.7% success rate on the MultiArith dataset, a 61 point increase over the last Google Researchers GPT-3 results with 17.7% (though keep in mind we used smaller models in our study).
- Model Size Matters: The effectiveness of CoT prompting is closely tied to the size of the model. Larger models (around 100 billion parameters) benefit the most, while smaller models might produce faulty reasoning or perform worse than standard prompting.
- Complex vs. Simple Tasks: CoT excels in handling complex problems but might not offer significant benefits for simpler tasks that require only one step to solve1.
- Transparency and Interpretability: By providing step-by-step reasoning, CoT makes AI’s decision-making process more transparent, which is crucial for applications in critical areas like healthcare, finance, and legal services.
- OpenAI’s o1 Model: The release of OpenAI’s o1 model has highlighted the importance of CoT prompting. This model has been trained to automatically use chain-of-thought reasoning, significantly improving its problem-solving capabilities across various domains.
- Multimodal CoT: Advances in CoT have extended its application to multimodal domains, where models can analyze visual cues alongside textual understanding to reason out detailed responses.
- Self-Correction: Recent research has shown that models employing CoT can self-correct, recognizing and correcting mistakes in their reasoning process.
Limitations and Challenges
- Resource Intensive: CoT prompting can be computationally demanding, potentially slowing down response times, which is a concern for applications requiring quick results.
- Overfitting and Bias: There’s a risk of overfitting or bias toward instructions introduced during instruction finetuning (IFT), as seen with ChatGPT, where it might implicitly follow CoT instructions even without being prompted.
- Limited Applicability: While effective for complex reasoning tasks, CoT might not be necessary or beneficial for simpler problems where direct answers suffice.